12023.
All that remains of our civilization is a curious movie dataset, found by aliens.
How will they imagine our world?
Dataset
Contextualization
Ten thousand years later and far away from our decaying home, alien explorers puzzle over the enigmatic treasure trove they have stumbled upon. Their advanced intellects delve into the intricacies of our stories, attempting to decipher the nuances of a civilization long gone. Through the lens of our cinematic legacy, these alien archaeologists seek to unravel the mysteries of what a human is. An alien student class decides to present what it has learned from the CMU Movie Summary Corpus to its teacher.
The Dataset
The CMU Movie Summary Corpus comprises 42,306 movies and an accompanying dataset featuring information about cinematic characters. The initial dataset, centered on movie details, encapsulates the narratives of 81,741 films spanning the cinematic landscape from 1888 to 2012. The second dataset complements this by presenting the specifics traits of 450,669 characters. In their first endeavor, the extraterrestrial scholars are poised to navigate through the character dataset. Using their preliminary analyses of these character details, they aim to extract significant insights into this species in order to draw a possible humanoid portrait.
Portrait
What does the typical human look like?
HELLO THERE! I’m R2D4, an inhabitant of the planet Xuluberlu. With my classmates, we are studying an archaic and extremely old civilization called “Humanity”.
Do you know what a typical human looks like? Bear with me, we are going to look at what our dataset has to tell us.
Basic physical descriptions
Gender
Let’s take a look at these two simple graphs.
Humanity clearly has two genders, but the distribution is quite unbalanced. The ratio evolves with time, but we should be able to say that there are around two times more males than females in this civilization. Maybe this is a genetic biais?
Age
I wonder how long humans lived. Do you think they had discovered a machine to extend their life expectancy? Let’s have a look at the actors’ age distribution.
75% of humans who played in movies are younger than 47 years old, and we observe that there are almost no actors older than 80. Apparently, humans had not discovered immortality. As well as dying young, these humans had a long education period. Only 25% of actors are under 28. If we now look at the breakdown by gender, we see a clear difference. There’s a median age difference of 8 years; does this mean that women have a shorter life expectancy?
Note to the human reader: In reality, the difference in life expectancy between men and women is very significant, it’s 5 years in the US but it’s all the way around, women have a longer life expectancy than men. .
Heigth
Come closer, reader! This is a representation of their heights. As you can see, they are not really tall (to give you an idea, one meter corresponds to a quarter of our medium size).
Again, there is a noticeable difference between men and women here.
Ethnicity
Now that we have an idea of their physical characteristics, let’s look at the last feature we haven’t exploited: ‘Ethnicity’. This might give us some information on where they came from and complete our physical description.
431 different ethnicities, that’s a lot! We cannot say that they all looked the same. The biggest category seems to be ‘Indians’, but I can see that there are a lot of smaller categories which have similar names, (Irish Americans, White Americans and Italian Americans). I wonder who those Americans are. Maybe I should continue my analysis by trying to group the small similar categories.
I wonder how you can fit such diversity in so tiny a planet: ‘Earth’.
What did they like?
Let’s move on from their external description. I now want to understand what they like, ie which movie genres they prefer. To do so, I need to have a way of quantifying how much they liked one movie over another. The column with the revenues for the movie box office seems perfectly suited for this. My unique worry is that this could be linked to other parameters which I’m not interested in. Indeed, I have just done a small linear regression on the movie box office revenue; these revenues seem to increase by a sum of $1 million for each year that passes. I definitely need to correct this effect if I want to have a reliable measure of movie success. Let’s assume that the distribution of successful and disastrous movies is somewhat similar through time. Based on that, we can independently normalize the movie box office for each movie release year. This brings the average to zero and is not very sensible to outliers. I introduced the feature called: ‘Movie box revenue scaled’, and I will assess the success of a movie based on the value of this measure.
Let’s start by looking at the movie genre distribution in the 1000 most successful movies.
The three main genres seem to be: Drama, Comedy and Action.
However, are we sure that those specific genres are able to induce success, or are we just seeing the most common movie genres? To be sure, I will show you a linear regression I made. To start with, I calculated the linear regression of the movie box office revenue variable against the movie genre. I chose the genre ‘Drama’ as a reference because it’s the most widely spread genre in the dataset and I looked at the influence of replacing the genre Drama by another one. Let’s take an example to be clear: a coefficient of 0.5 associated with the genre ‘Computer Animation’ means that having the genre ‘Computer Animation’ versus having the genre ‘Drama’ brings you a success of 0.5 points higher. I show here only a subset of the genre we assessed.
Looking at this, three genres stand to outperform ‘Drama’: Glamorized Spy Films, Computer Animation and Roadshow theatrical releases. On the contrary, the genres: Gay, Experimental and Gay interest seem to be very detrimental to a movie’s success.
This is only the result of the comparison against the reference ‘Drama’, to be complete I need to compare them with other references. After having tried all the different genres as reference I can tell you that those results are very robust. When I tried sequentially to use all the genres as references I see that it’s always the same three that outperform almost all the other genres and the same three that underperform. This means that the results are very robust and we maybe have found this the key to grasp human preferences.
However, to be fully complete, I need to ensure that I’m really looking at the influence of the movie genre on the success of the movie and not the influence of other confounders. To avoid the influence of the confounders it’s convenient to do some pair matching, the matching algorithm used outputs a dataset balanced between the two genres we are investing in which movies share similar languages and countries of origin. By doing so, we correct for the influence of those two parameters.
For Computer Animation movies and Drama we can see that the result holds, Computer Animation is significantly better (with a p-value inferior to 0.005) than Drama. However, it’s not the case for all of them. If I can also ensure that Glamorized Spy Film is better than Drama I cannot say anything about the others, indeed, for them, the p-value associated with the linear regression coefficient in the pair matching is higher than 0.005.
How do they work?
Military ranks
Some character names give us precious information about human occupations, especially using their denomination. Let’s focus on the character names which begin by a military rank (around three percent of them! (2.7%)).
Note to the human reader: three per cent of military personnel is a lot, comparatively to World Bank data on December 2023, for which less than 0.5% of the total world population (around 30 million people) are military personnel.
We get the following graph.
Since we know the most graded personnel is also the scariest one, I guess the previous graph gives us a good approximation of the hierarchy of ranks in the army: a “Captain” is, therefore, less graded than a “Private”.
Note to the human reader: poor R2D4! He couldn’t know that he is victim to a terrible cofactor here: the interest of a given military rank for movie scenarists. Indeed, it is more likely to see a Captain than a Private on the silver screen, not because there are fewer Privates than Captains, but because a Captain has so many more responsibilities than a Private, and therefore is more interesting for scenarists.
Doctor positions
I was reading our data, when I noticed a singular fact: lots of characters are called ‘Dr.’.
Whoooah, such a miss! A t-test (p-value < 1e-59) discredits the null-hypothesis “There are as many female doctors as female actors in general”.
Note to the human reader: the bias in female representation in movies is a well-known gender effect.
Conclusion
Well, this journey into the CMU movie dataset was very insightful. I hope that you now have a clearer idea of what a modern human looked like back in the year 2000 when this species still existed. It seemed to be a small, quiet species from one of the furthest reaches of the Milky Way. This species was made up of two rather poorly distributed genders. Humans had a rather moderate size and had a life expectancy of around 80 years old. Their main language was undeniably English, although their ethnic origins were extremely diverse. Concerning their tastes, they seemed to be very sensitive to Glamorized Spy Film and Computer Animation. When I have time, I will take a closer look at the content of plot summaries to better identify what drives human curiosity in those two genres. Here, I sketched the ID card of the average human from the CMU movie dataset.
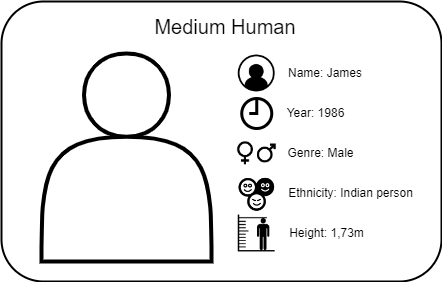
Map
The Map
After delving into what makes a robot portrait, this inquisitive extraterrestrial explorer, (me!), decided to delve into a dataset and craft a map of Earth. Leveraging insights from my alien cousin in the Star Wars galaxy, I speculated that the “Movie countries” in the movie dataset might correspond to places where humans dwell on our planet. In my cosmic imagination, Earth took the form of a whole planet without water (well I don’t know what water is, but the human writing my story does), and I envisioned countries as points on its surface where human activity is concentrated. I believe that by scrutinizing links between countries sharing movies, I can unveil connections. Simplifying the links to one dimension—represented by the number of shared movies—made the analysis more manageable.
In my model, each graph edge symbolizes this one-dimensional link between countries. Drawing on my knowledge of network data, I transformed the movie countries into an adjacency matrix, forming a graph where countries become nodes and shared movie links are transformed into edges. Each link is weighted by the number of movies shared. Before sketching the graph, I pondered how to model the attraction between countries. My extraterrestrial mind toyed with the idea of paralleling it to gravity, envisioning a force that pulls two countries towards each other. Firstly, I decided to assess the importance of each node by creating its degree, unveiling the crucial nodes. Then, delving into more intricate measures, I explored Katz centrality and Eigenvector centrality. While both PageRank and Katz centrality are designed for directed graphs, my creation turned out to be undirected. With no other choice, I opted for Eigenvector centrality as a means to compute the importance of a node in the graph. A high eigenvector score, I realized, would indicate that a node is intricately linked to many others, each holding substantial importance themselves in the vast cosmic network I’ve constructed.
From my extraterrestrial perspective, the Earth map unfolds as a mesmerizing display, skillfully depicting the intricate connections that weave through the planet. As I observe, the countries appear closely situated, revealing noteworthy relationships among them with a deep core interconnection.
To facilitate understanding, the map incorporates one circle just for the sake of visualization.
Note to the human reader: R2D4 doesn’t know that actually he is seeing in its deep core the G12 plus three countries. That’s why I allowed myself to add a second circle to highlight the G12 countries. The G12 is an informal group of 12 countries that are the 12 largest economies of the world and this plot highlights that they influence in a way the world, a way part of the soft power.
Names
What to say about human names?
Assumptions for the first names part
The first part presents the analysis made by R2D4 in which he established a human-robot portrait using the dataframe characterDF
, the question we can ask ourselves now is what are the aliens looking at. What is the quality of the data, Is it a fair representation of human societies and more fundamentally, is the dataset of movies only a description of human society or rather something which also has the power to influence social trends? To perform this analysis we are going to look at human first name distribution and we are going to compare the name distribution in the CMU dataset and in the USA.
From this second dataset, it will be possible to compare the first name distribution over the years and see if the movie dataset is representative of reality. It could also help us see if a first name trend arrives in the movie dataset before real life or vice versa.
For this purpose, we have to define the rules:
- The study will be done on the USA population as the baby name dataset is only for this country.
- The study will be done on first names that are present in the movie dataset with a movie box office revenue.
- We will consider a valid first name if the first name is present IN the character name (it could be its first name or its last name or even a middle name).
Creation of new first names in birth register that come from the top 100 movies
When we extract the first name from the character name, we first conduct a simple comparison to know if some first names are present in movies before the baby name dataset. We found that 84 first names have a first appearance in a movie before being given to a baby.
Just by hovering with a mouse over the above treemap, one can see the movie where the first name appears for the first time, as well as the first year when this first name was given to a baby. Among the 84 first names, it is impossible to conclude that all of them were given to a baby due to the movie (e.g., despite Star Wars being an influential saga, one cannot conclude that the name Emperor given in 2008 is due to Star Wars V released in 1980). However, it could be the case for others, and it is interesting to see from which film the name comes.
Examples of three first names where the movie is more likely to have created the first name which was given to a baby after the movie’s release:
- Bellatrix (Harry Potter and the Order of the Phoenix, 2007)
- Neytiri (Avatar, 2009)
- Samwise (The Lord of the Rings: The Fellowship of the Ring, 2001)
Correlation of first name trends in movies and in birth registers
Now armed with a dataset from their extraterrestrial professor, our alien students can eagerly explore Earth’s first name trends in movies and in the USA birth register. He is interested in seeing if the trends are the same or not. This way, he could see if the first name distribution in the movie dataset is representative of reality over the years.
After data exploration and correct work on the dataset, the alien was ready with his dataset. He filtered the dataset for first names that come from a movie that has a value in the movie box office revenue column and computed the normalized frequency from both datasets.
For the sake of clarity of what is now in his dataset before conducting a deeper analysis, the alien student decides to plot the normalized frequency of some arbitrarily chosen first names in movies and in the birth register. The line about the movies is smoothed by the savgol filter.
As the alien student can notice from this graph, some trends are sometimes different (in advance or delayed). Thus, he thinks that doing a linear regression won’t help him to evaluate this delay; it could only assess the correlation between the two trends. He decides to do a cross-correlation analysis to see if there is a delay between the two trends. He computes the cross-correlation between the two trends for each first name and plots the distribution of the delay. During the cross-correlation, the alien applied a weight based on the score of the movie (the higher the movie box office revenue, the higher the weight).
In simplifying the graph to highlight the top 200 first names for clarity, our diligent alien student discerns a notable feature. Comparing it to the previous graphs with the two curves, the alien observes that cross-correlation effectively pinpoints the time delay between the curves. This proves especially adept when both curves showcase a single peak. However, the alien notes a nuance in cases where the time delay is highly negative. In these instances, the time delay value lacks coherent interpretation because the two curves exhibit strictly opposing trends, making accurate peak comparison challenging.
As the plot is not simplified with all first names, the alien student decides to plot the time delay for all first names on a scatter plot and on a log scale. This way, he can see the distribution of the time delay for all first names.
Carefully noting that a time delay of 0 is predominant, there’s a suggestive indication that, in general, first names align with the trends observed in movie first names. This prompts a fascinating hypothesis: people may name their babies with the influence of the names they encounter in movies. However, it’s crucial to approach this correlation cautiously. The lack of consideration for potential confounders in this interpretation demands prudence. Without accounting for various influencing factors, prematurely concluding a causal link between movie trends and baby names may lead to misconceptions. The alien student, maintaining a vigilant stance, refrains from definitive conclusions, recognizing the need for a nuanced understanding of Earth’s naming patterns.
To enhance the alien investigation, he suggests to his alien teachers to incorporate datasets on potential confounders, such as major events (e.g., Olympics, elections), celebrity influences, and local factors. However, disentangling these complex influences poses a significant challenge.
Set of movies
Which set of movies do we have to send to the aliens to accurately represent Earth?
Summary of the strategy
From now on, we leave the point of view of aliens, and adopt that of scientists on Earth who aim at elaborating the optimal movie dataset to send to space, so as to represent the Earth accurately. How to find the best pool? And how many movies shoud this pool contain? To answer these questions, we begin with defining a metric that assesses scores of pools of movies. We then optimize the number N_opt that should be sent. We can now iteratively construct our optimal pool. This enables us to analyze the sensitivity of our scoring method. We also use machine learning methods such as linear regressions and random forests to assess the influences of movies genres on the scores.
- Definition of the metric
The metric is composed of 4 subscores: parity, diversity, height, and age. Further details about how we built it can be found in the notebook.
Let’s have a quick look at the following scatterplot matrix. It shows the distributions and relationships between the four subscores. What we see is that each distribution is close to a gaussian function, which is good in order to find some existing pools significantly better than the average. Also, the very low values for R^2 reveal that the subscores have relatively low linear dependencies between one another. There might be unmastered confonders, but their eventual effects seem weak. The only two scores that appear to have a correlation are parity and height. Though it is easy to associate it with the fact that more men usually leads to a higher average height among the actors, that should not be the explanation. Indeed, we already took this fact into consideration by separating between male and female height distributions in our scoring function. Thus, the correlation is hard to explain.
- Why do we assess the scores of pools of movies and not of movies?
A movie alone may have not enough actors to be representative of society. It also may be about a particular event, place, era, that does not describe comprehensively our society (wars under the Roman Empire for instance). It may even be about science-fiction. For these reasons, we have concluded that scores over one movie are likely to be very insignificant. This translates into a fairly high variance when we look at the distribution of scores over pools of one movie only.
- Then, is there an optimal number N_opt of movies per pool?
The following tradeoff occurs: if N increases, the variance of the scores of K pools of N movies should decrease. But on average, the film industry is not representative of society, with obvious biases in gender and ethnicities. Therefore, an increase in N should result in increases in the bias.
The picture above reveals the tradeoff. The red curve (the average spread in amplitude of our score) decreases with N. We would like it to be large, to discriminate effectively for great pools. The blue curve (inverse of variability of the mean score of 1,000 pools) increases with N. We want it to be high, so that the variablility remains low between two random distributions. As a consequence, we choose a value of N that is not too detrimental to either of the criteria we consider.
We choose N = 20 movies per pool as of now. We can notice that the average number of actors per movie is around 8. This means that for 20 movies, we will do statistics over about 160 actors. This seems sufficient to be somehow representative.
- How to find the optimal pool of 20 movies?
The strategy here is not to find the 20 best scores. This would be disproportionately complex. We rather apply the following idea. First, we create 10,000 random pools of 10 films, and keep the best pool. Thanks to this, we can have much higher scores than what we had with 20 movies (scores around 85 vs 75 before). A t-test reveals that such scores are critically unlikely if we compare with random distributions of pools of 20 movies. From this basis, we iterate over all remaining movies to find the one that most improves the total score. We then have 11 movies, and redo the exact same thing, until the pool is composed of 20 movies.
Using this method we obtain the following pool of 20 movies with a representativeness score of 89 (much more than the 75 we had before). The movies are:
- Running Mates
- Blackball
- North by Northwest
- Cool Hand Luke
- Son in Law,
- My 5 Wives
- Try Seventeen
- A Nightmare on Elm Street 3: Dream Warriors
- Paradise Road, Kicking & Screaming
- Zany Adventures of Robin Hood
- Super Sweet 16: The Movie
- Vipers
- State’s Evidence
- Twelve Monkeys
- Exorcist II: The Heretic
- Trial and Error
- Panic
- Recess: Taking the Fifth Grade
On the way to a bigger pool? We chose to use $10$ movies with a high score to begin, because, in the other case, the computational cost is too high. Interestingly, the goal of our algorithm is to keep the excellent score of our initial pool, rather than improving it. Since the 10 additional movies are here to maintain the diversity of the pool, they are chosen to be the best in their respective category, so we can hope to get a mega dataset by taking these representativeness-conserving movies, and amazingly (also manually…) we obtained a pool of 60 movies having a score of 82 ! This is quite exciting because pools of that size normally have a maximal representativeness score that is around 50. Still, this has a severe computational cost.
- Is our model sensitive to changes in the scoring function?
A very arbitrary parameter we chose is the list of weightings that we attribute to each subscore, to sum up to the total score. What happens if this weighting is modified? Does an optimal pool for a previous scoring remain good? For this purpose, we define 6 different weightings, and evaluate the scores of these 10 pools on each new weighting.
Results:
We observe that each pool has worse scores for all weightings other than the initial one. However, the scores remain in the range of excellent scores, so they are no longer the optimal pool. This translates the fact that our metric is not too one-sided but rather takes every subscore into consideration.
- Machine learning tools:
The goal is to assess the influence of some genres on the score of pools of movies. We decide to focus on the genres that appear in at leas 10% of the movies of our filtered dataset. This lets us with mostly Drama, Comedy, Thriller, Action Adventure, and other broad categories (10 genres in total). We simulate 10,000 random pools of 20 movies. For each, we build features that are equal to the frequency of each of these genres in the pool. We also give 5 features that should help the models fit the scores: mean and standard deviations of actors’ ages and heights in the pool, and parity in the pool.
We separate our 10,000 pools into 8,000 pools for training and 2,000 for testing. In order to assess whether genres influence scores or not, we perform analyses thanks to linear regression and random forests, both with all the features, and then without the genres. The following graphs show the results for the random forests model with and without the genres.
The difference in standard deviation of the distributions of predicted scores between both seems negligible: 14.08 (with genres) vs 14.63 (without). A t-test also gives a very small difference in their average values but a great p-value (about 0.95).
For now and in regards to the studies we have conducted, we conclude that the genres we have studied do not influence the accuracy in predicting scores.
Conclusion
Conclusion
December 19, 2023, 11:23 AM: Voyager 1 has ceased responding.
After 46 years of dedicated service, this spacecraft is now adrift in the vastness of space. As of today, it holds the distinction of being the farthest object ever crafted by human hands, positioned 2.436563e+10km away from Earth. Onboard, scientists have left a message intended for any extraterrestrial beings that may encounter it –– a gold-plated audio-visual disc known as the famous golden disk. This disc features a selection of symbols, images, and sounds designed to encapsulate and represent our world. The looming questions persist: Will these alien entities understand the message? Will they gain insight into our world and, by extension, into us as a species?
With our dataset, R2D4 the alien has extracted the portrait of an average inhabitant of planet Earth in the early 2000’s using only the CMU movie dataset. This includes a physical description of humans and an analysis of their tastes. Was it representative of reality? That’s what we tried to investigate by looking at the distribution of jobs and names in the dataset; it appears that the cinema industry is not exactly close to reality. In order to avoid inducing aliens in error (which is a very mean thing to do) we tried to extract a subset of representative movies which could be used by aliens to have a more realistic vision of humanity. Will this suffice? Only the future will tell…